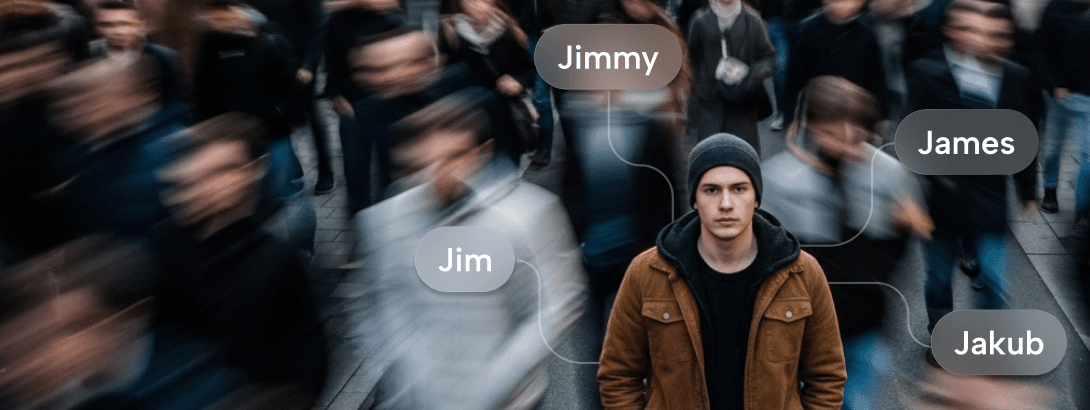
Why LLMs are the new standard in watchlist screening
For years, compliance teams have been forced to treat false positives as an unavoidable tradeoff. You could catch bad actors or you could avoid endless alert queues but not both.
That mindset no longer holds.
Today, bad data is more than a nuisance. It creates regulatory risk, slows down onboarding, and undermines confidence in compliance operations.
One Watchlist customer told us:
“We’re not just overwhelmed by false positives. We’re being embarrassed by them.”
That’s what one compliance exec told us before switching to Socure’s watchlist engine. Their system was misidentifying E*TRADE Bank as Myanmar Foreign Trade Bank. Ion Bank was flagged for a sanctioned entity in Beirut. “International Bank” triggered alerts tied to the Russian Federation.
These weren’t edge cases. They were constant. And they were costing teams time, credibility, and trust.
The Stakes Are Climbing
Regulators have taken notice.
- TD Bank paid $3B after allowing over $470M in illicit flows
- Metro Bank missed £51B in questionable transactions
- Starling Bank faced a £29M penalty over PEP screening failures
In parallel, fraud tactics are getting smarter:
- Shells & Complex Ownership: PEPs hide behind multi-layered corporate structures.
- Aliases & Transliterations: “Aleksandr” becomes “Alexander,” and most systems miss the match.
- Stale Watchlists: If lists aren’t constantly updated, you miss designations that happen post-onboarding.
- Synthetic Docs: Fake identities are increasingly hard to detect without document and biometric validation.
Barclays learned this the hard way – £72M in fines tied to a £1.88B transaction involving high-risk PEPs.
Old methods aren’t cutting it.
A New Engine for Screening: LLMs with Context
We rebuilt our watchlist engine from the ground up using Large Language Models (LLMs) trained not just on characters, but on meaning.
Traditional systems use string matching. Ours doesn’t stop there.
Socure’s LLMs evaluate:
- The structure and semantics of names
- Subsidiary and parent relationships
- Spelling, spacing, transliteration, and cross-lingual variants
- Cultural naming conventions and name order
- Reference chains across text (e.g. resolving “she” to “Jane” with high confidence)
Our distributed architecture runs a network of fine-tuned LLMs. Each is specialized for speed and precision. The result: 80ms latency and 500 TPS – fast enough for real-time use, accurate enough for regulators.
What That Looks Like in Practice
- 98%+ accuracy in name degradation testing (vs ~80% for others)
- False positive ratio improved from 100:1 to 3:1
- 100% recall during testing across PEP/sanctioned individuals
- 20x reduction in false positives per 1,000 checks
- Review time dropped from 10–15 min to 2.5 min per alert
- 0 tier-2 escalations due to misflags of known institutions in past 90 days
Compliance teams get fewer escalations. Business teams stop second-guessing alerts. Analysts get time back.
How We Measure Performance
- Precision: Of the alerts we surface, how many are real?
- Recall: Of the real threats, how many did we catch?
- False Positive Rate: How much noise are we generating per 1,000 checks?
- Review Time: How long does it take an analyst to clear a match?
What’s Next
We’re expanding the same LLM-based logic to identity match scoring – linking fragmented records to answer the question: Is this the same person or just a similar name?
We’re also retraining our regression models to improve explainability for risk-based decisions. That means faster tuning, clearer outcomes, and less reliance on manual QA.
And because regulators are demanding transparency, we’re prioritizing:
- Model validation
- Traceability
- Audit-ready logic
Our models are explainable, auditable, and structured for regulator review supporting obligations under BSA, OFAC, and FATF guidelines.
Smarter Screening Starts Here
Compliance doesn’t need to come with compromise.
With Socure’s LLM-powered watchlist engine, you don’t have to choose between catching threats and cutting false positives.
You can do both. With precision.
Talk to us about ditching string-matching. Your compliance team deserves better.
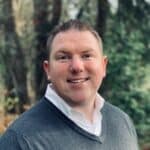
Josh Linn
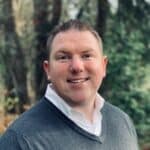
Josh Linn
Related Posts
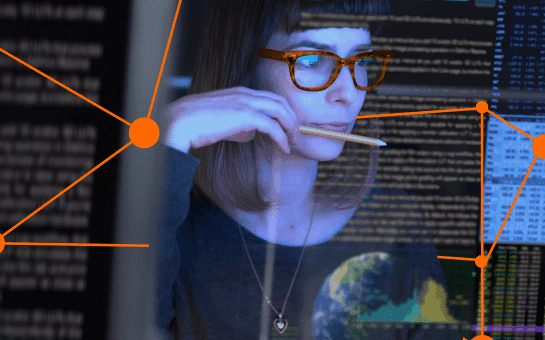
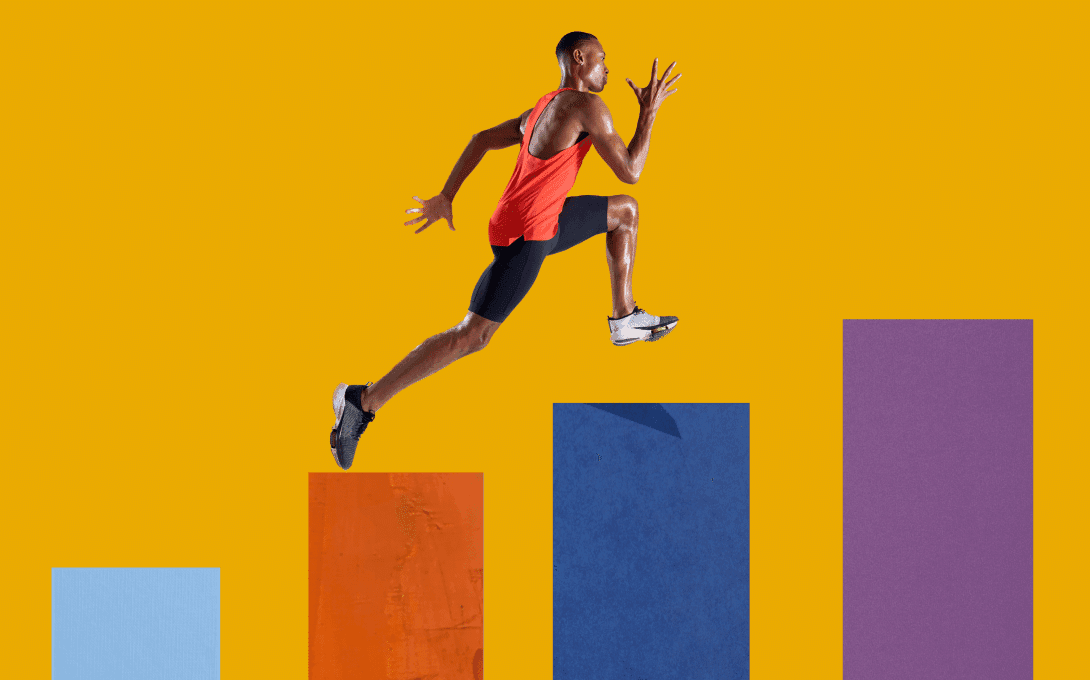
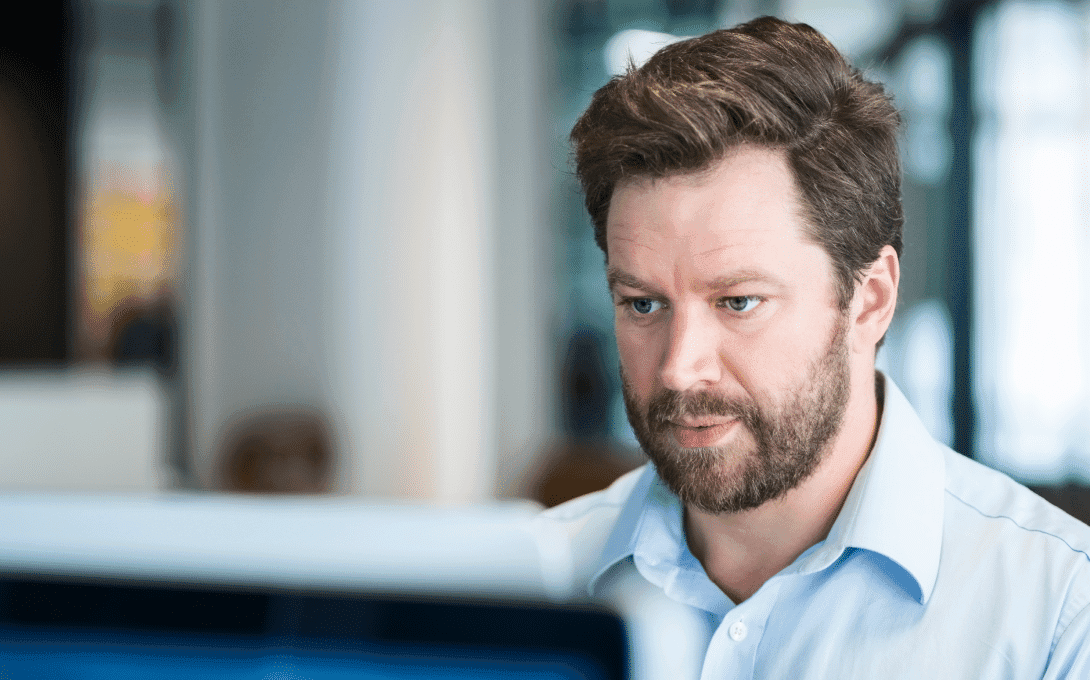