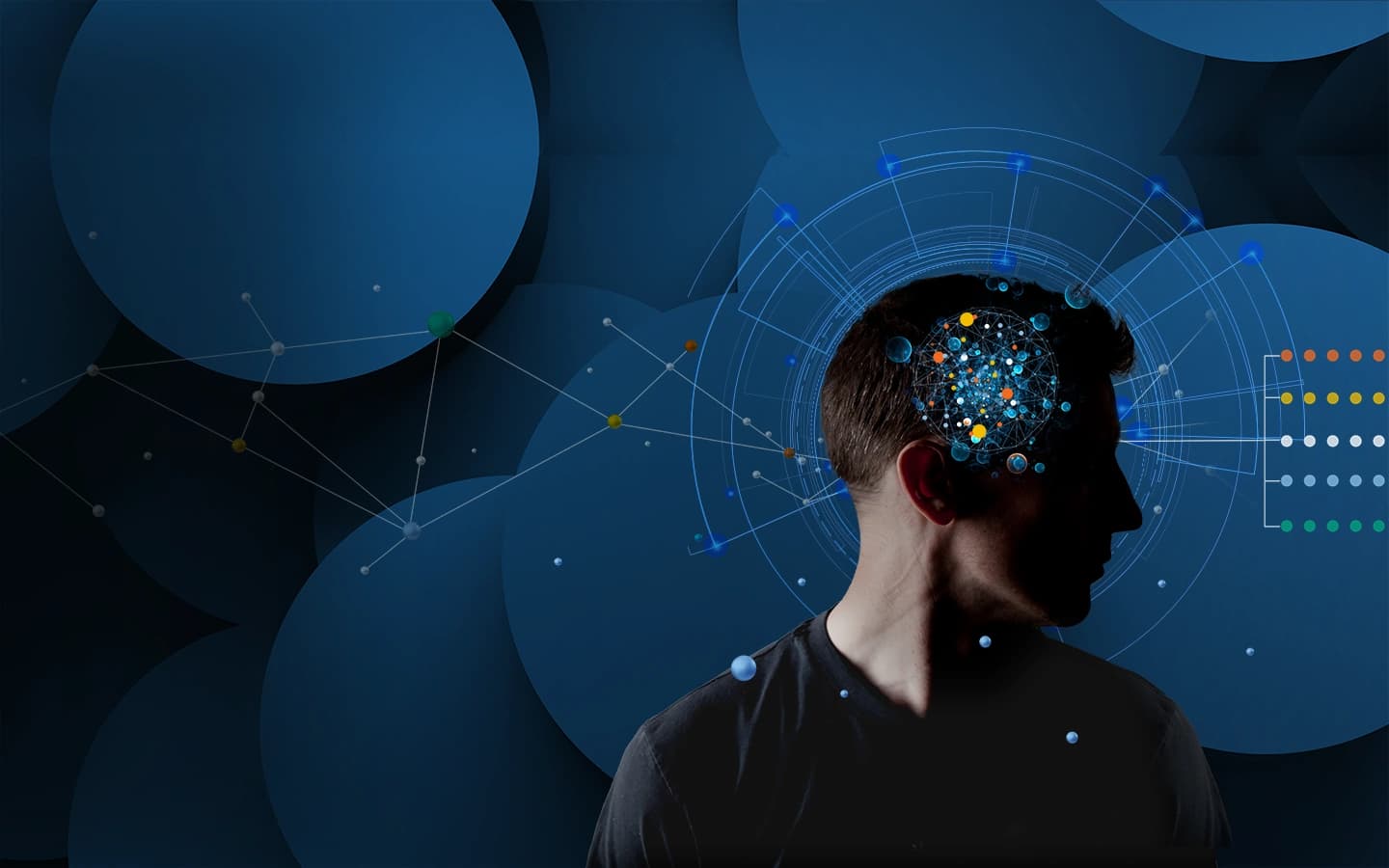
Smarter Synthetic Identity Fraud Detection
Sigma Synthetic Fraud uses advanced machine learning and diverse, high-quality third-party and historical data to uncover complex patterns and connect identity elements associated with synthetic identity fraud, so your organization can capture fraud at the door — making way for more good customers.
Synthetic Identity Fraud Patterns are Constantly Evolving — No Industry is Safe
As consumers grow their digital footprints across devices, channels, and geographies, differentiating malicious synthetic behavior from that of good consumers is harder than ever. Traditional rules-based systems and third-party fraud solutions fall short in solving this pervasive fraud.
Without the benefits of a consortium network, your fraud investigation team has a limited ability to identify tricky elements of synthetic identity fraud, exposing your business and customers to unnecessary risk.
The Socure Difference
Socure’s powerful AI has been trained for more than a decade on 400+ third-party data sources and cross-industry network feedback from 2,700+ customers that fight fraud together. The world’s largest organizations in financial services, government, gaming, e-commerce, and other industries lean on Socure’s Sigma Synthetic Fraud solution to proactively identify risk and protect their businesses — in real time.
Benefits
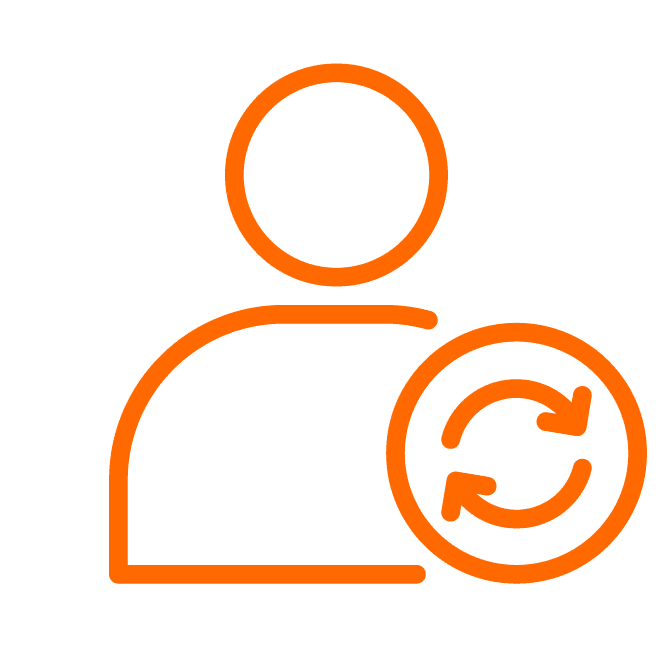
Employ proactive identity fraud detection
Employ proactive identity fraud detection
Predict and prevent synthetic fraud before it happens to avoid financial losses
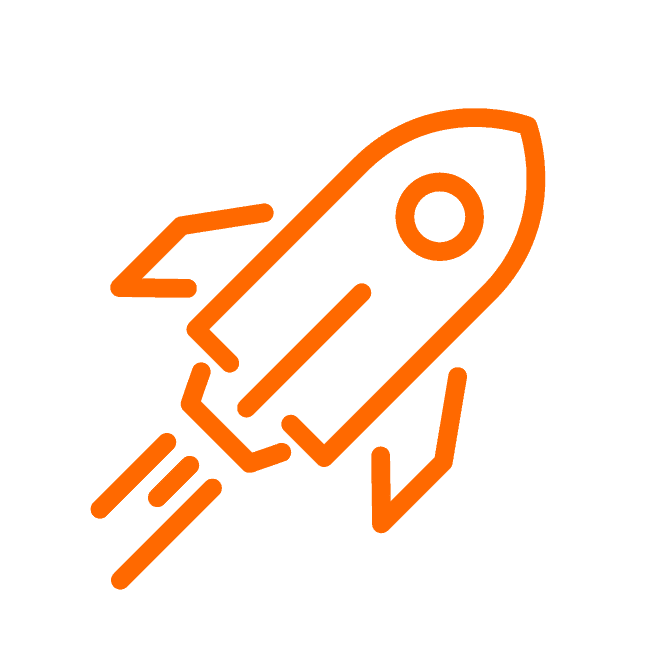
Improve user experience
Improve user experience
Reduce false positives and boost user satisfaction with quick and reliable approvals
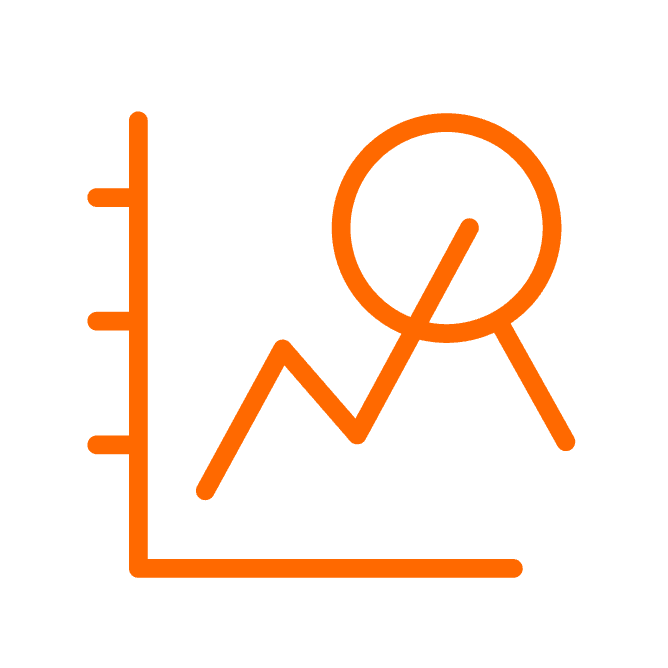
Open more good accounts
Open more good accounts
Drive revenue growth by auto approving more good customers faster
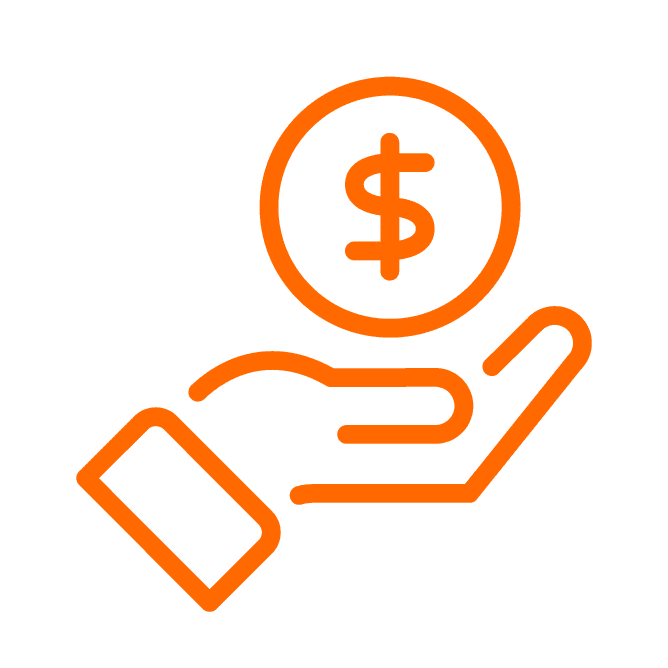
Lower operational costs
Lower operational costs
Reduce manual reviews and operational expenses with a fully automated solution
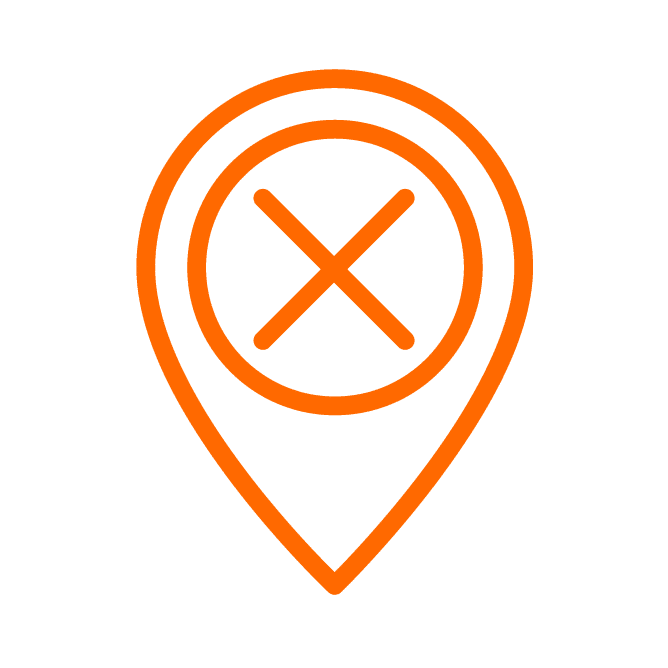
Prevent reputational damage
Prevent reputational damage
Avoid enforcement action and fake account prosecution
Solution Highlights
Target fabricated and manipulated identities
The Sigma Synthetic Fraud model applies the Federal Reserve’s definition of synthetic identity fraud which focuses on “manipulated” and “fabricated” types to support customers in determining the optimal follow-on treatment — without adding unnecessary friction.
Access 400+ third-party data sources
Real-time risk detection analyzes credit header, credit inquiry, proof of life, and hundreds of other data sources, combined with velocity intelligence across diverse segments to detect anomalous synthetic patterns.
Leverage a powerful consortium database
A consortium network of more than 2,700 diverse customers enables Socure to understand identity elements and patterns to build a robust database of 2 billion good and bad identities — bolstered by 150 million outcomes added in the past year.
Outsmart synthetics with AI that thinks like a fraudster
Ingesting cleanly-labeled feedback data back into the model trains the system to think like a fraudster and accurately predicts and detects evolving synthetic fraud threats.
Calculate Sigma Synthetic Fraud Score
The Sigma Synthetic model returns a predictive fraud score that gauges identity risk calculated on thousands of synthetic-specific features, linkages, and patterns.
Employ interpretable technology
Simple-to-understand Reason Codes with each Fraud Score provide explainable insight to empower decisioning.
Let us prove it.
See how you can achieve world-class results with Socure. Speak with a digital fraud expert to learn more about our synthetic identity fraud tool and ask us about also employing the full Sigma Fraud Suite to solve first-party fraud and third-party fraud challenges.