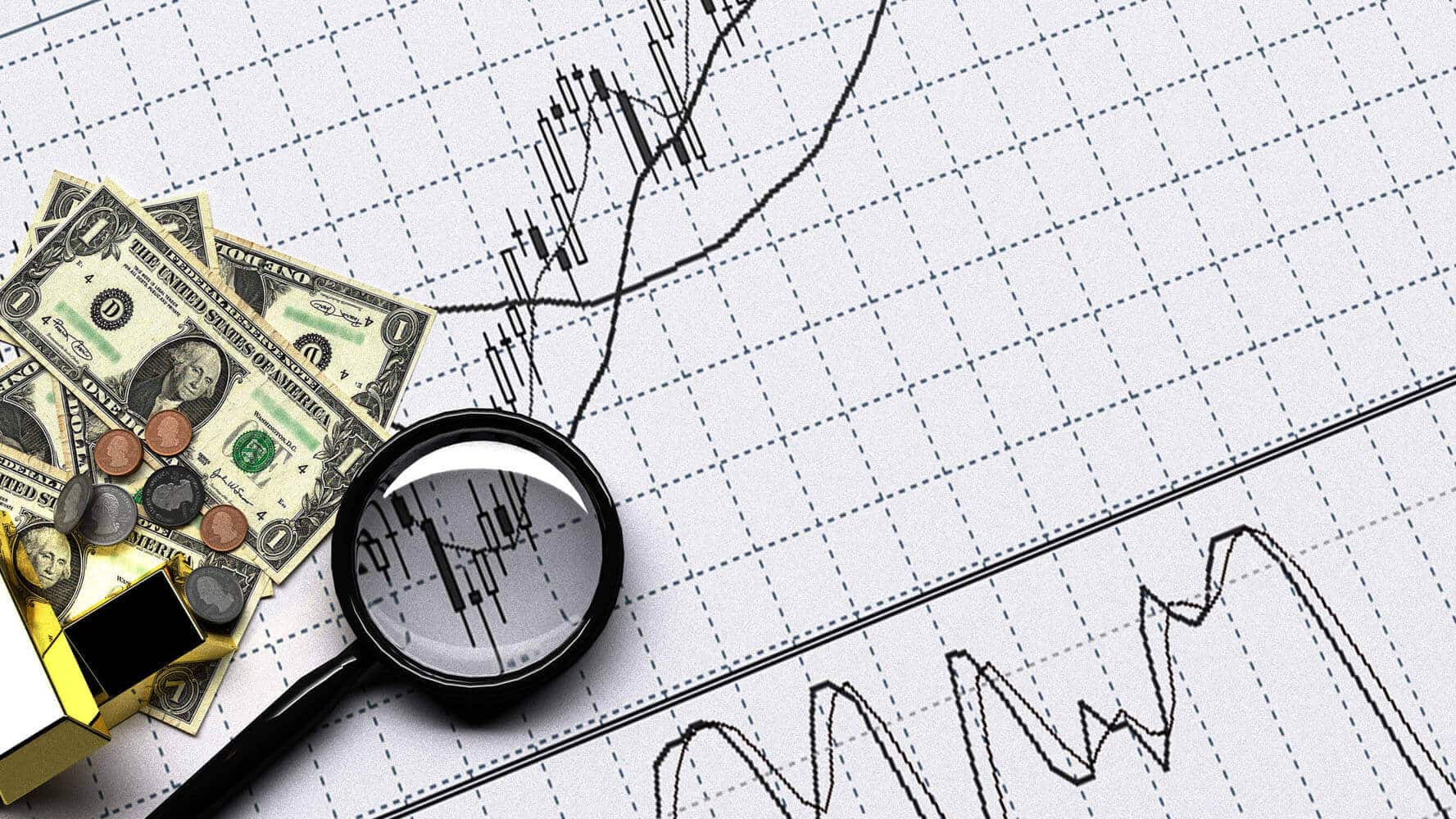
December 14, 2020
Protecting Your Financial Products from Identity Fraud: ‘You don’t have to run faster than the bear’
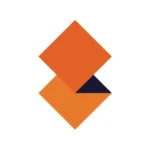
Socure
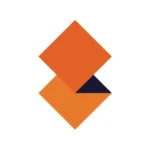
Socure
Socure is the leading platform for digital identity verification and trust. Its predictive analytics platform applies artificial intelligence and machine learning techniques with trusted online/offline data intelligence from email, phone, address, IP, device, velocity, and the broader internet to verify identities in real time.
Related Posts
Addressing the Role of Synthetic Identity in P2P Fraud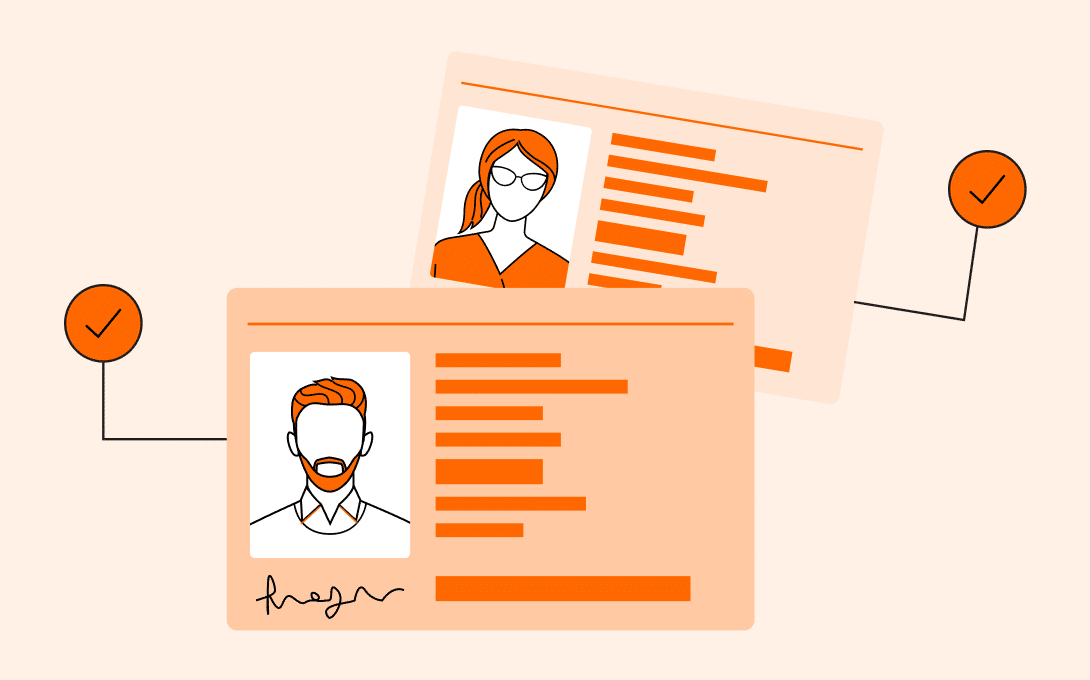
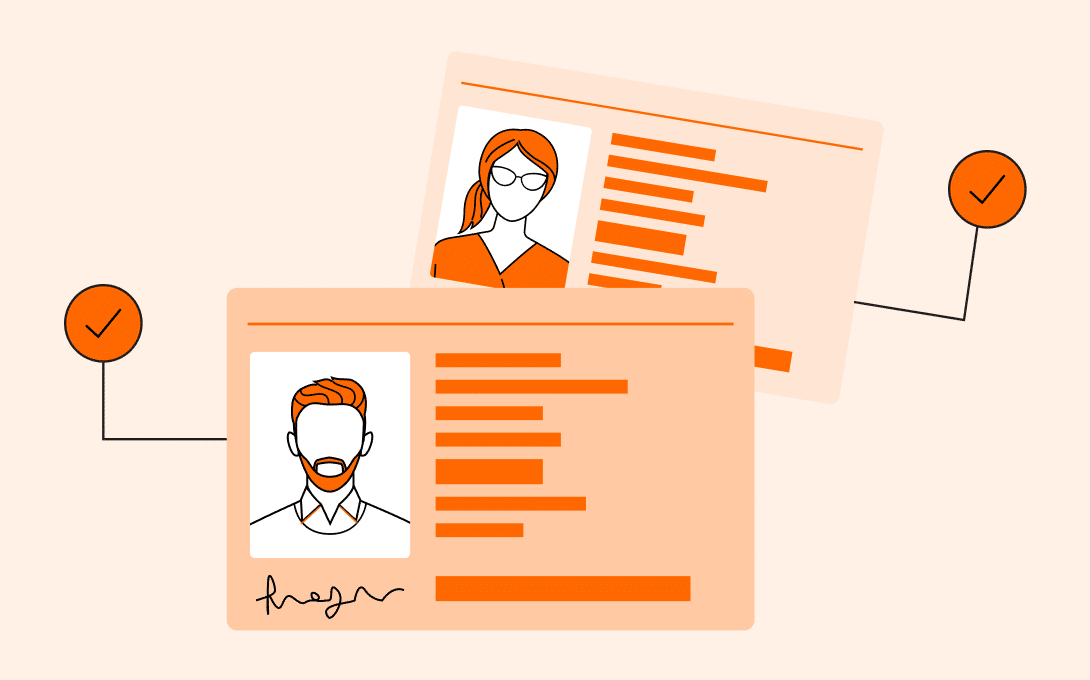
December 13, 2022
Addressing the Role of Synthetic Identity in P2P Fraud
‘Tis the Season for Fraud Attacks: Banks, Credit Card, and BNPL Hit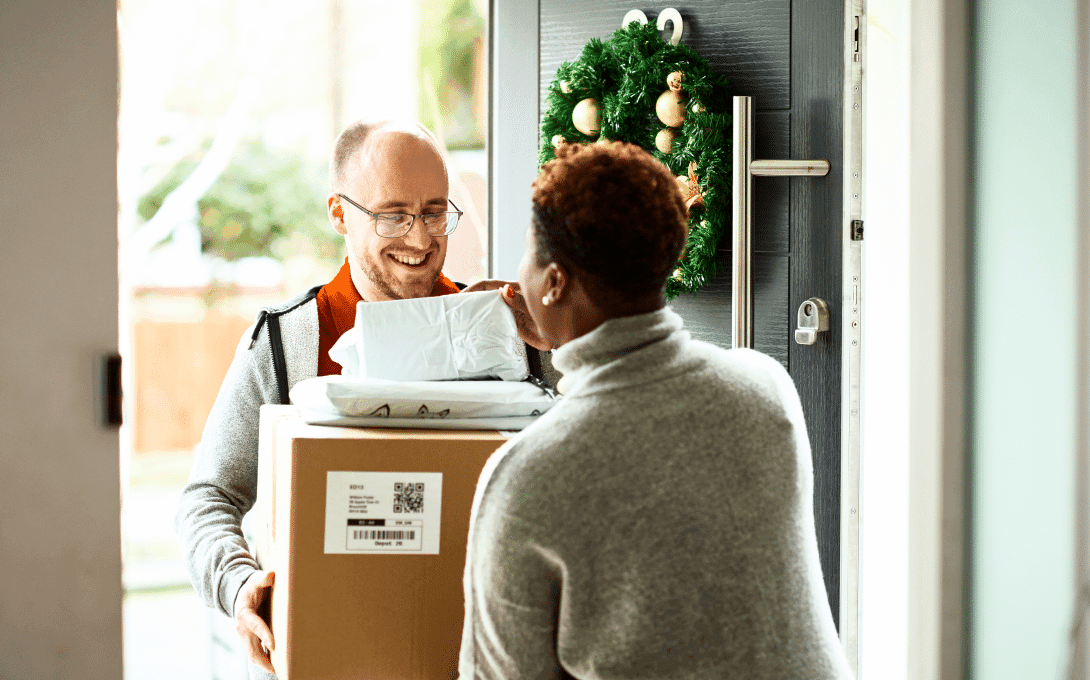
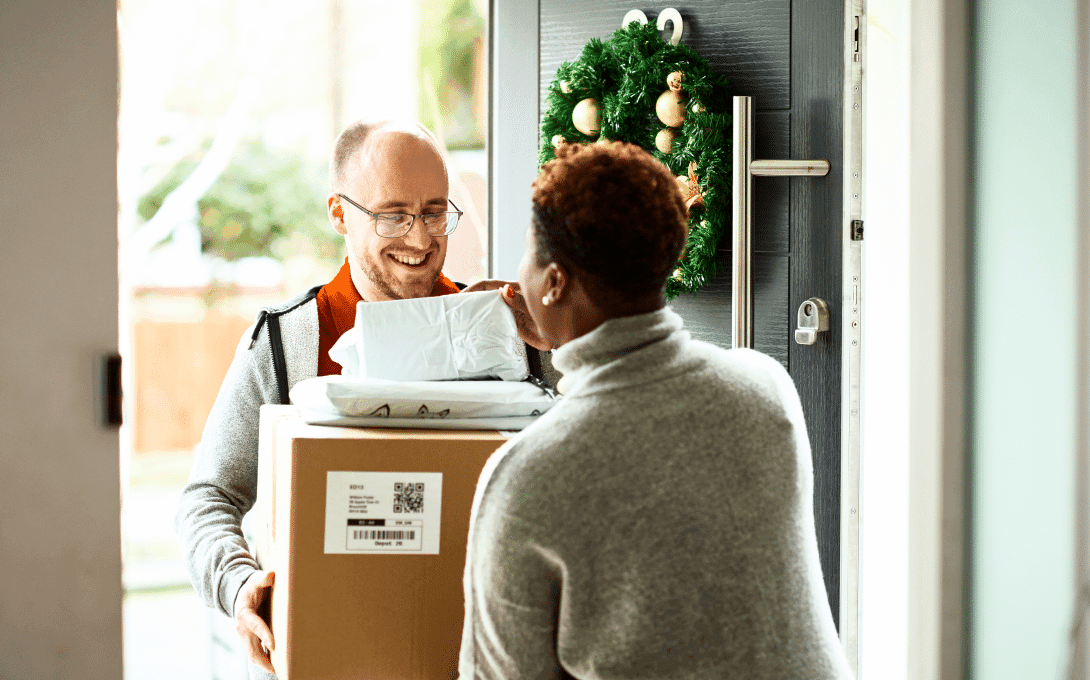
December 07, 2023
‘Tis the Season for Fraud Attacks: Banks, Credit Card, and BNPL Hit
3 Common Digital ID Verification Pain Points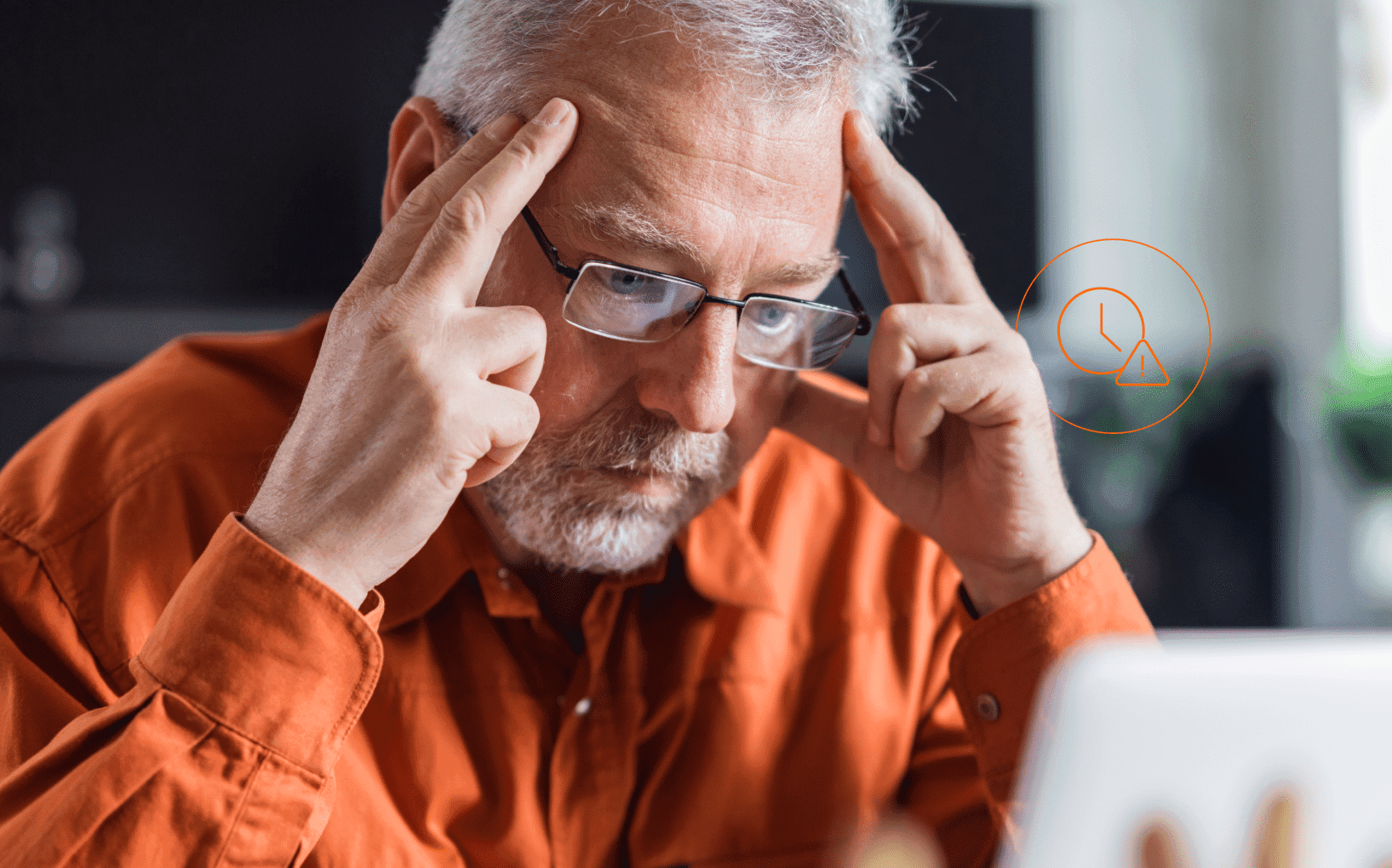
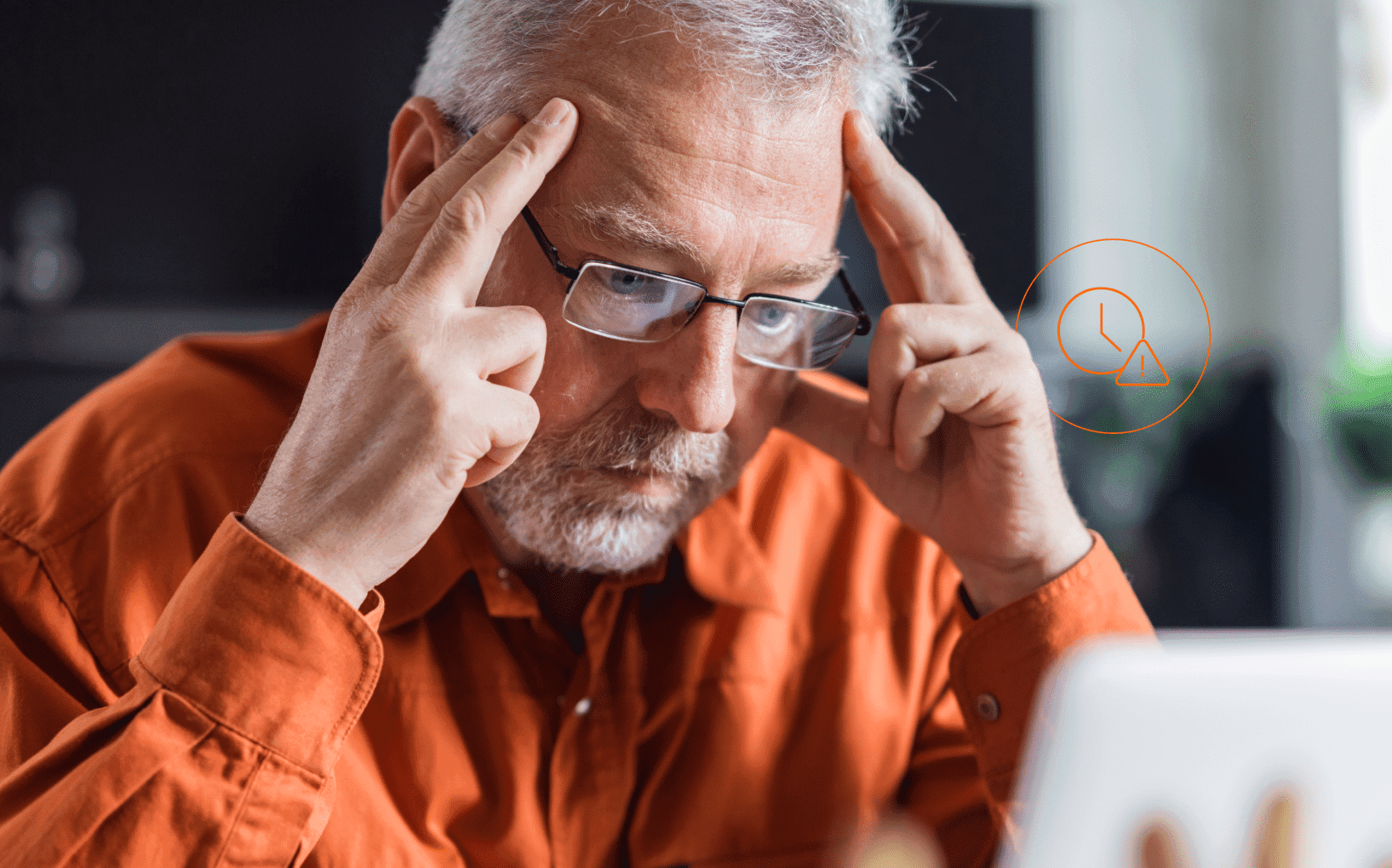
February 14, 2022
3 Common Digital ID Verification Pain Points