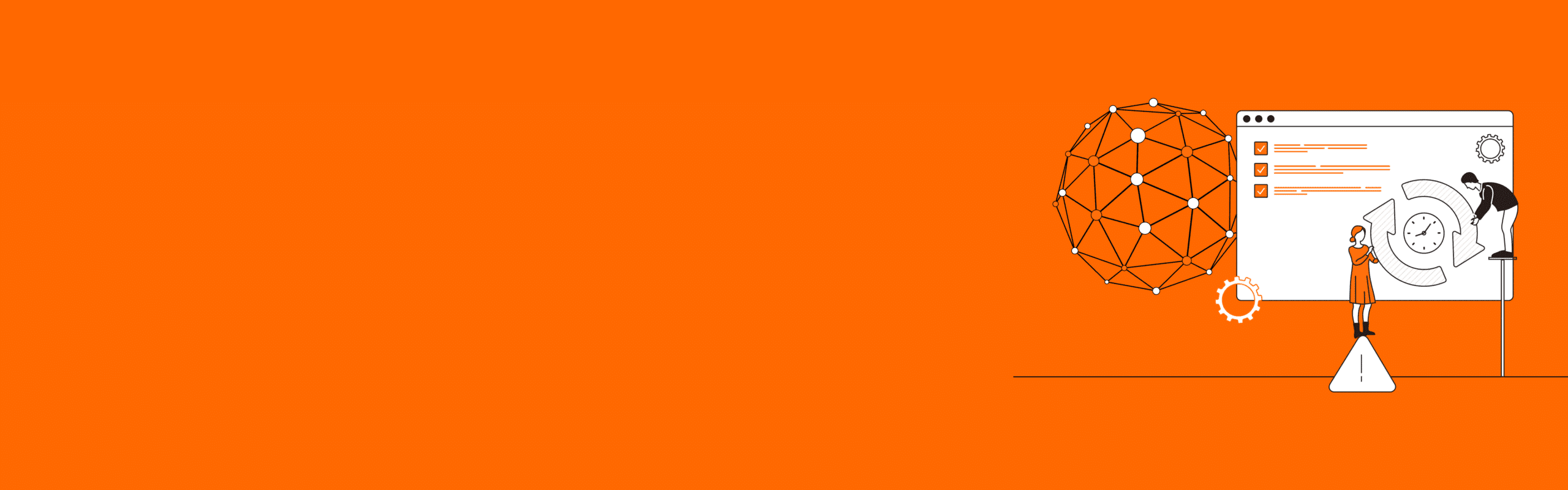
November 02, 2023
The Power of Machine Learning for Better Compliance Outcomes
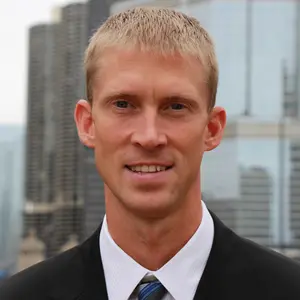
Matt Johnson
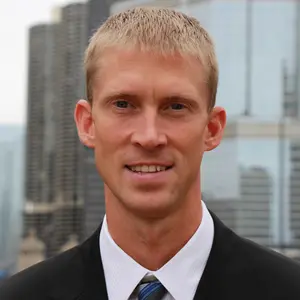
Matt Johnson
Matt is the Director of Product Marketing for KYC and Global Watchlist solutions at Socure. Prior to Socure, Matt established and led the product marketing efforts for fraud and identity solutions at TransUnion.
Related Posts
New Federal Plans for Artificial Intelligence Must Be Paired With Digital Identity Implementation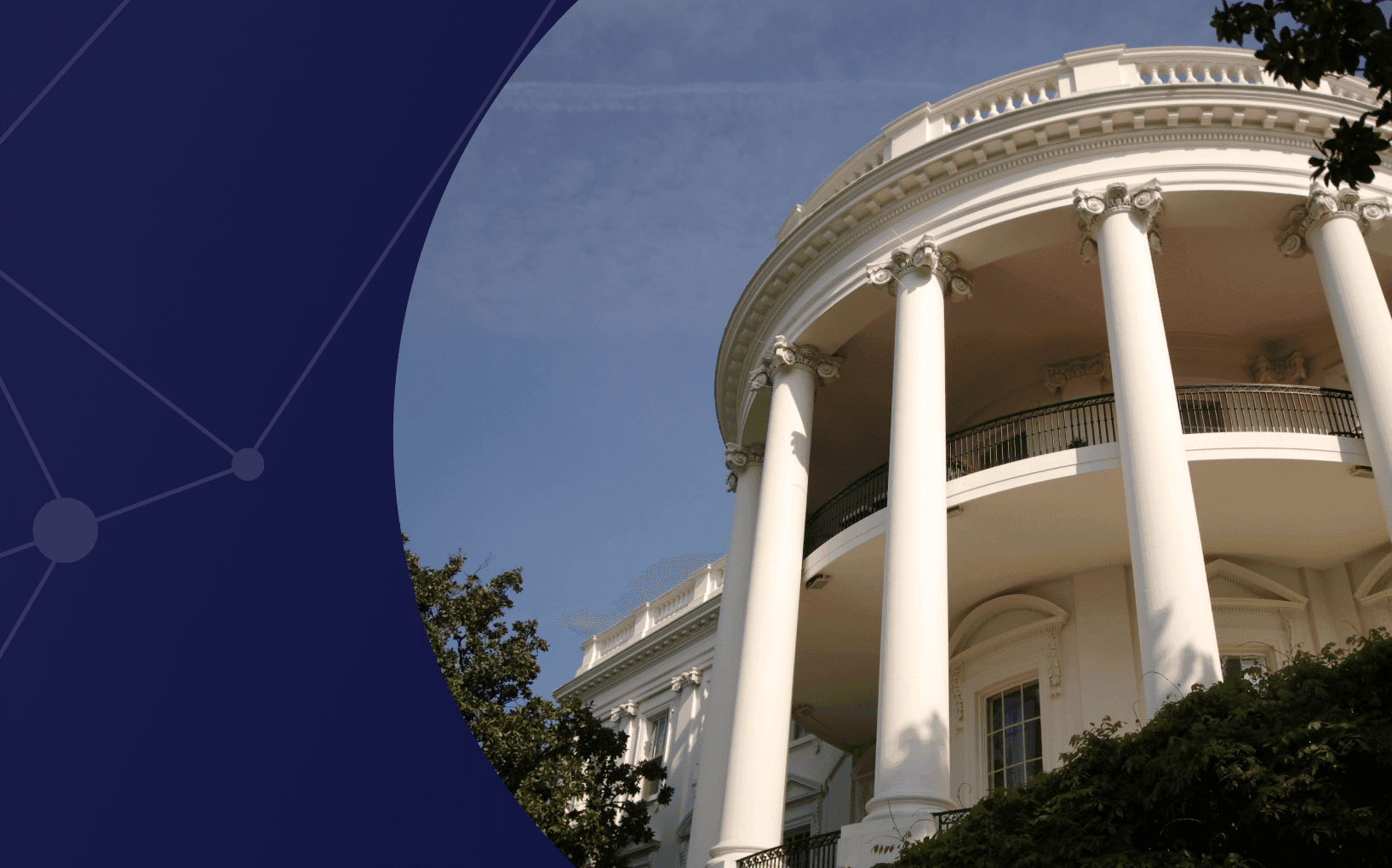
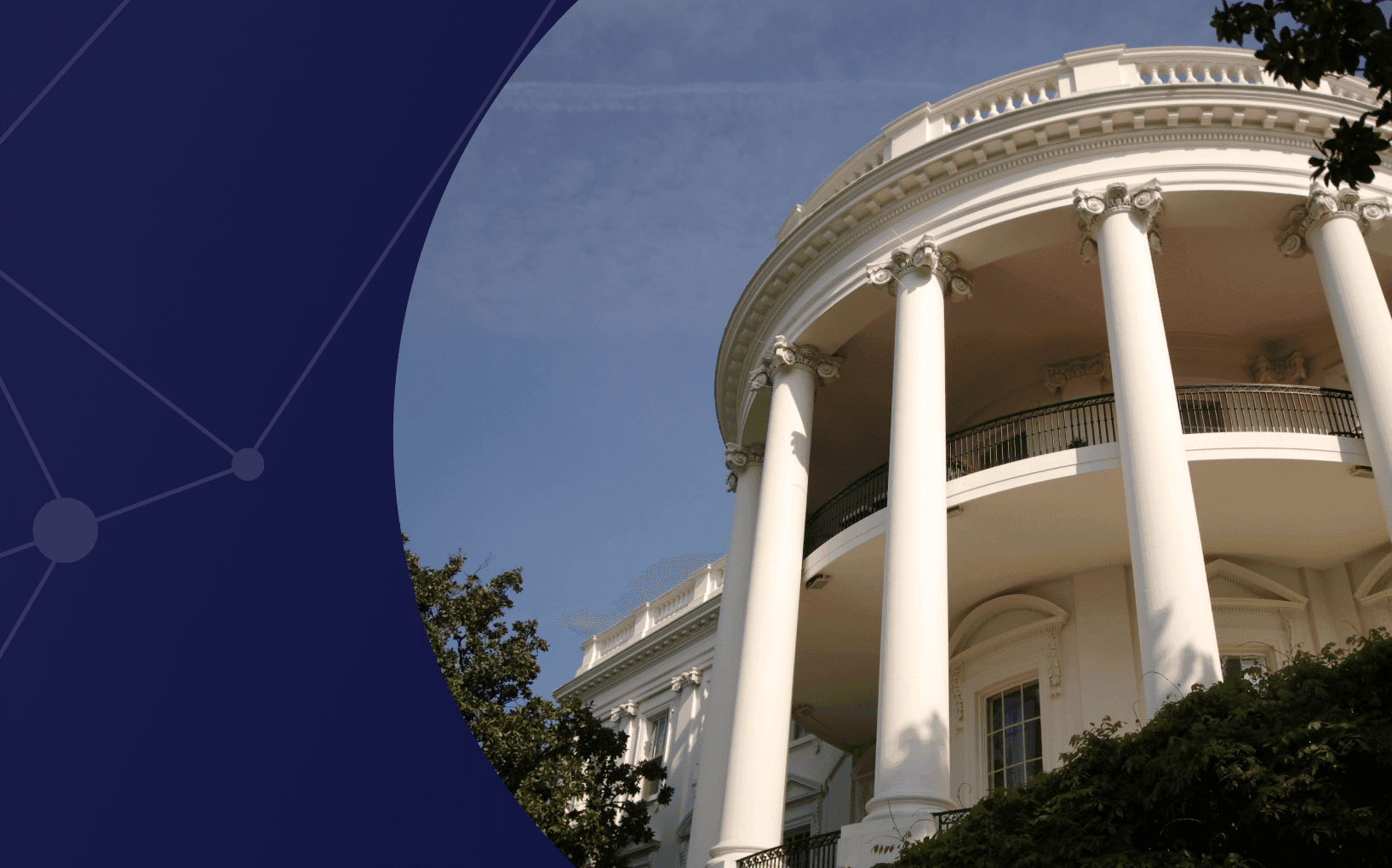
October 30, 2023
New Federal Plans for Artificial Intelligence Must Be Paired With Digital Identity Implementation
Uncovering Hidden Threats: Why Auditing Your Portfolio for Money Mules is Critical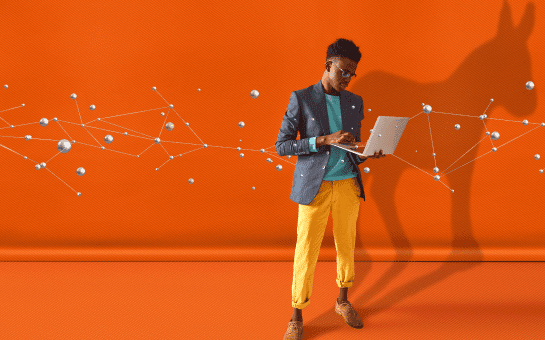
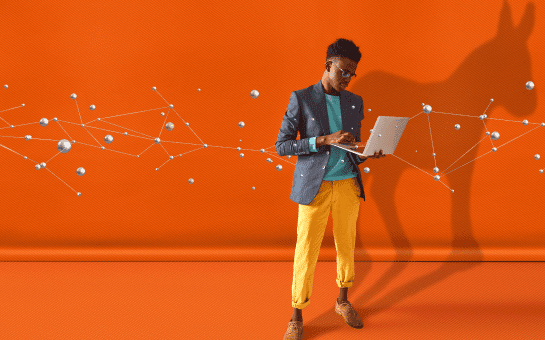
July 28, 2023
Uncovering Hidden Threats: Why Auditing Your Portfolio for Money Mules is Critical
Socure Solution Insights: Expanding Coverage and Improving Precision with the Latest Advances in Deceased and Address Data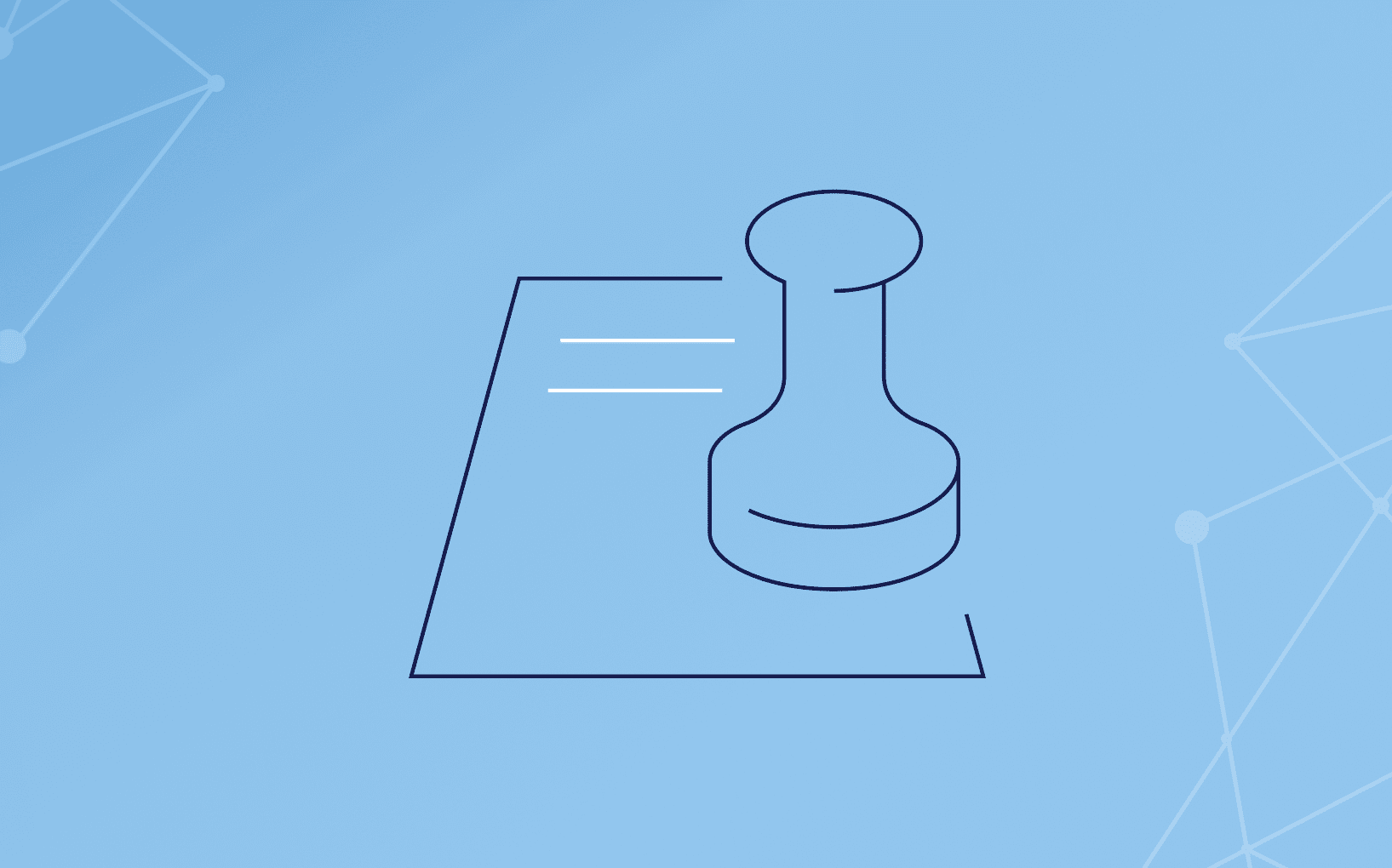
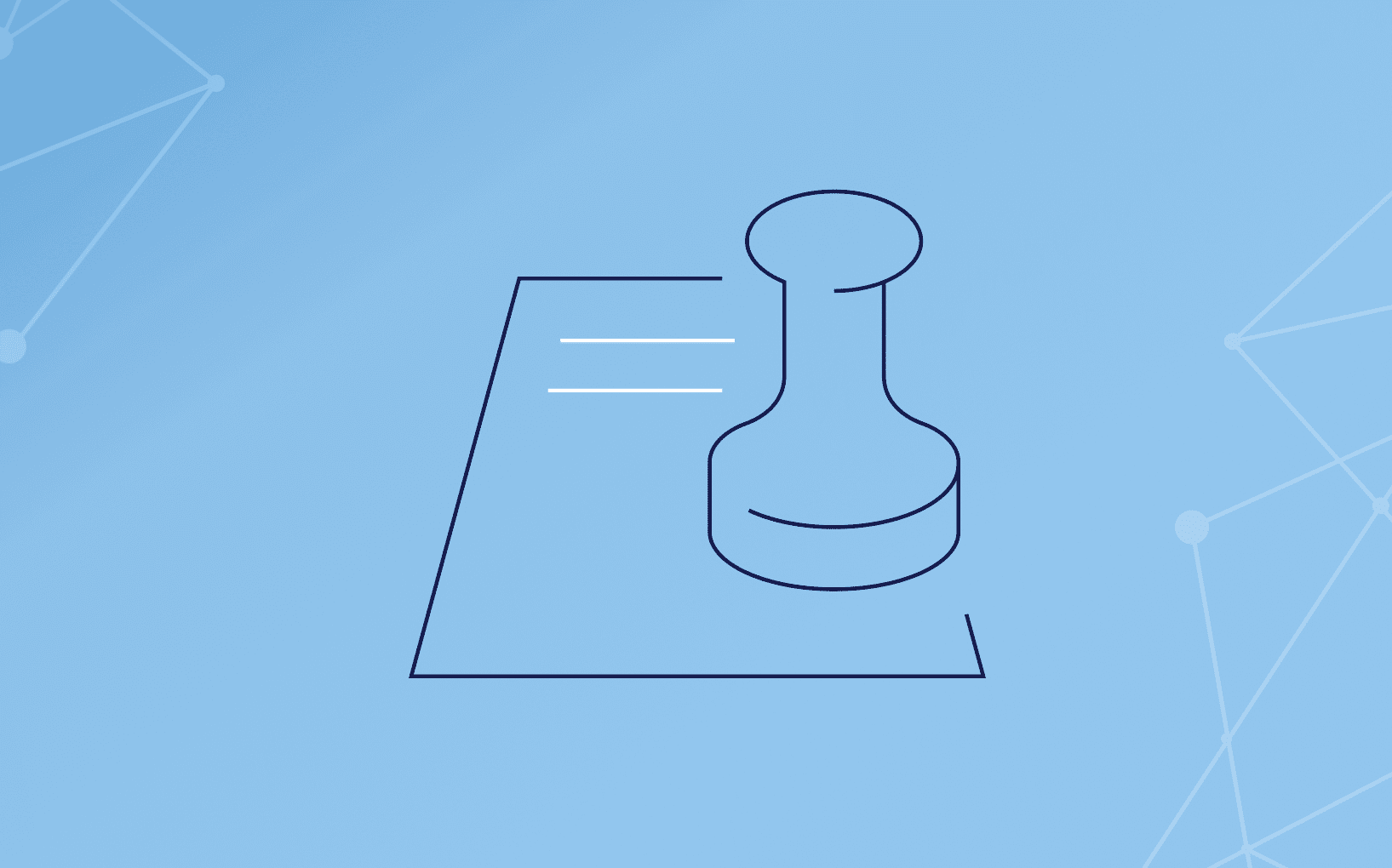
September 25, 2023
Socure Solution Insights: Expanding Coverage and Improving Precision with the Latest Advances in Deceased and Address Data