
The Real Identity Blog
Socure’s Real Identity Blog is the best source to learn about identity verification and fraud decisioning trends, news, and best practices.
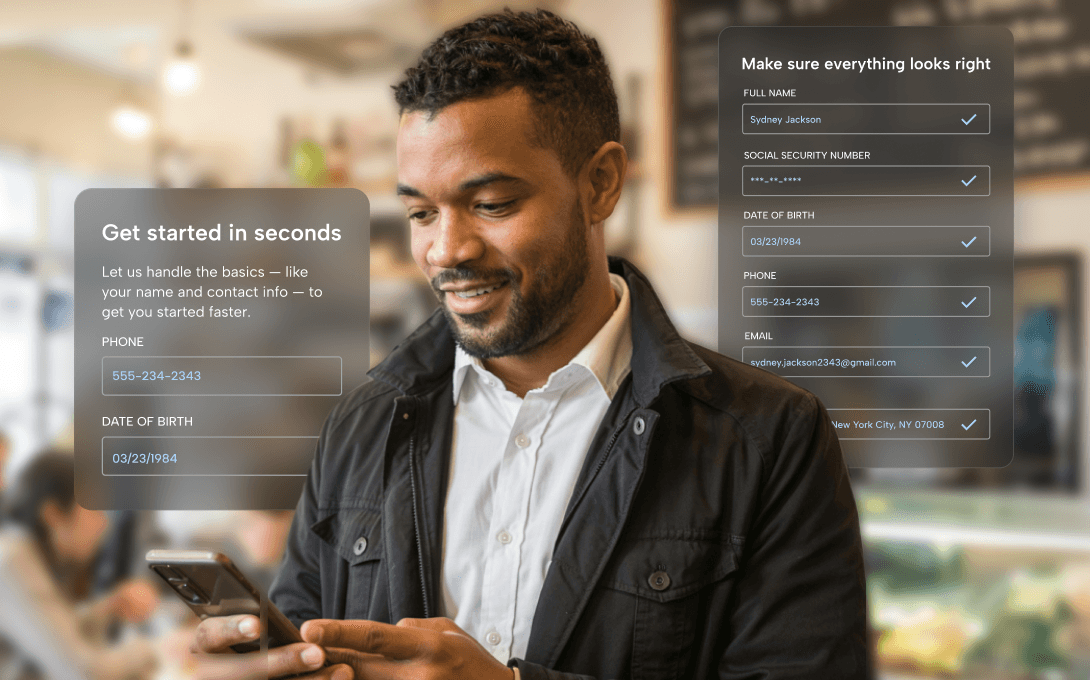
Featured
Introducing Socure Advanced Pre-Fill
Socure’s suite of products and use cases consistently lead the market in the areas of Identity verification, fraud prevention, and risk decisioning. How? The answer is simple – and profoundly powerful: It all starts with the Socure ID graph.
Filter By:
All topics
Loading...
Let us prove it.
Join the 2,800+ top enterprises that trust Socure. Speak with one of our identity verification and fraud experts today.