
The Real Identity Blog
Socure’s Real Identity Blog is the best source to learn about identity verification and fraud decisioning trends, news, and best practices.
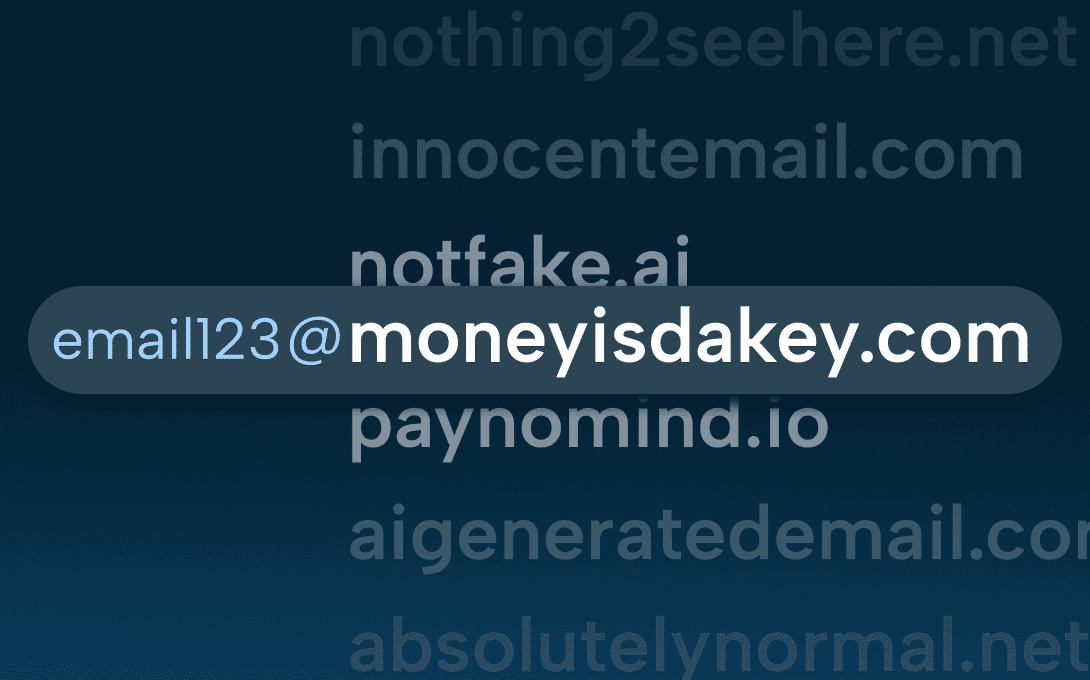
Featured
The Shape-Shifting Domains of Digital Fraud Rings
Fraud doesn’t wear a mask — sometimes it wears a URL. And more often than not, that URL is registered through a domain registrar you’ve never heard of, hosted on a server in a country you’ve never done business in, and abandoned before anyone notices it existed.
Filter By:
All topics
Loading...
Let us prove it.
Join the 2,800+ top enterprises that trust Socure. Speak with one of our identity verification and fraud experts today.