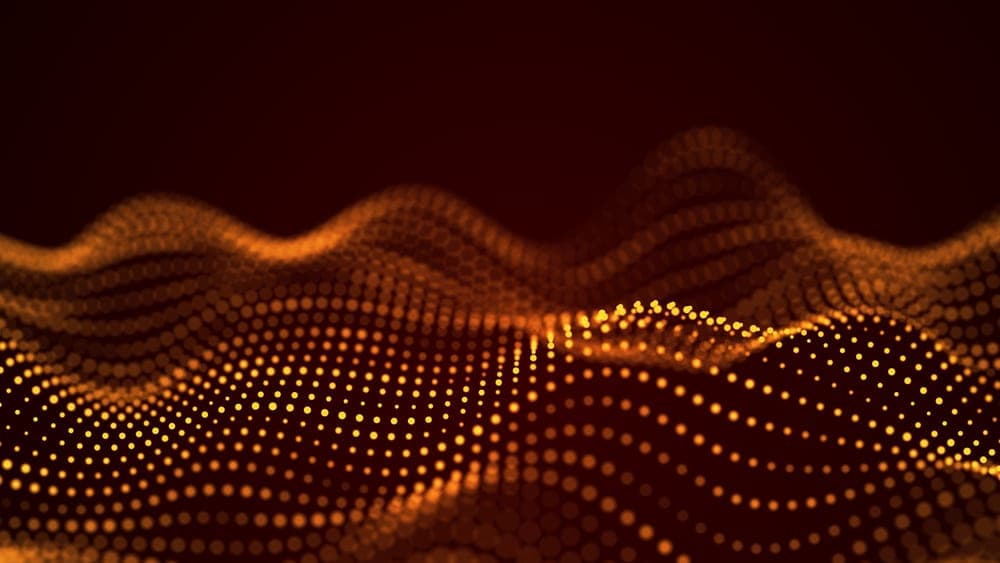
July 11, 2022
Aite Report Highlights Socure’s Graph-Defined Approach to Synthetic Identity Fraud
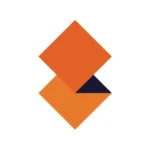
Socure
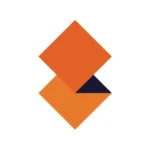
Socure
Socure is the leading platform for digital identity verification and trust. Its predictive analytics platform applies artificial intelligence and machine learning techniques with trusted online/offline data intelligence from email, phone, address, IP, device, velocity, and the broader internet to verify identities in real time.
Related Posts
Synthetic Fraud Q&A with Socure’s Founder & CPO Johnny Ayers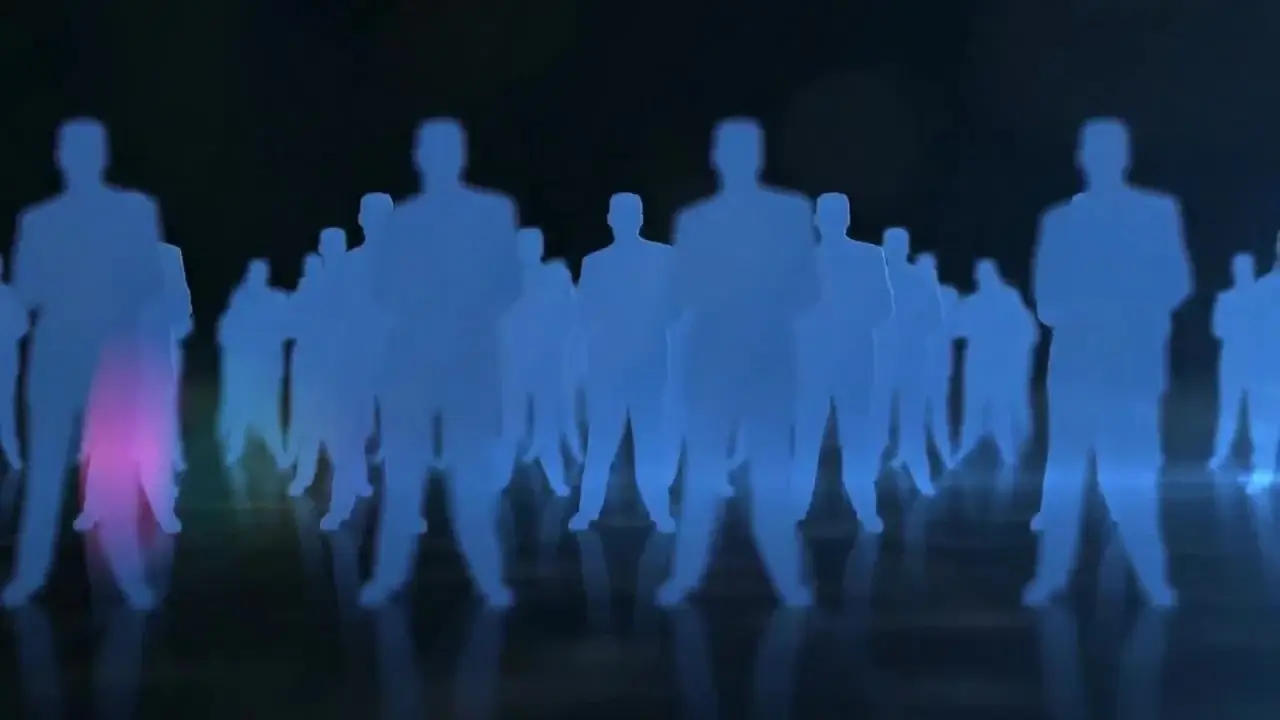
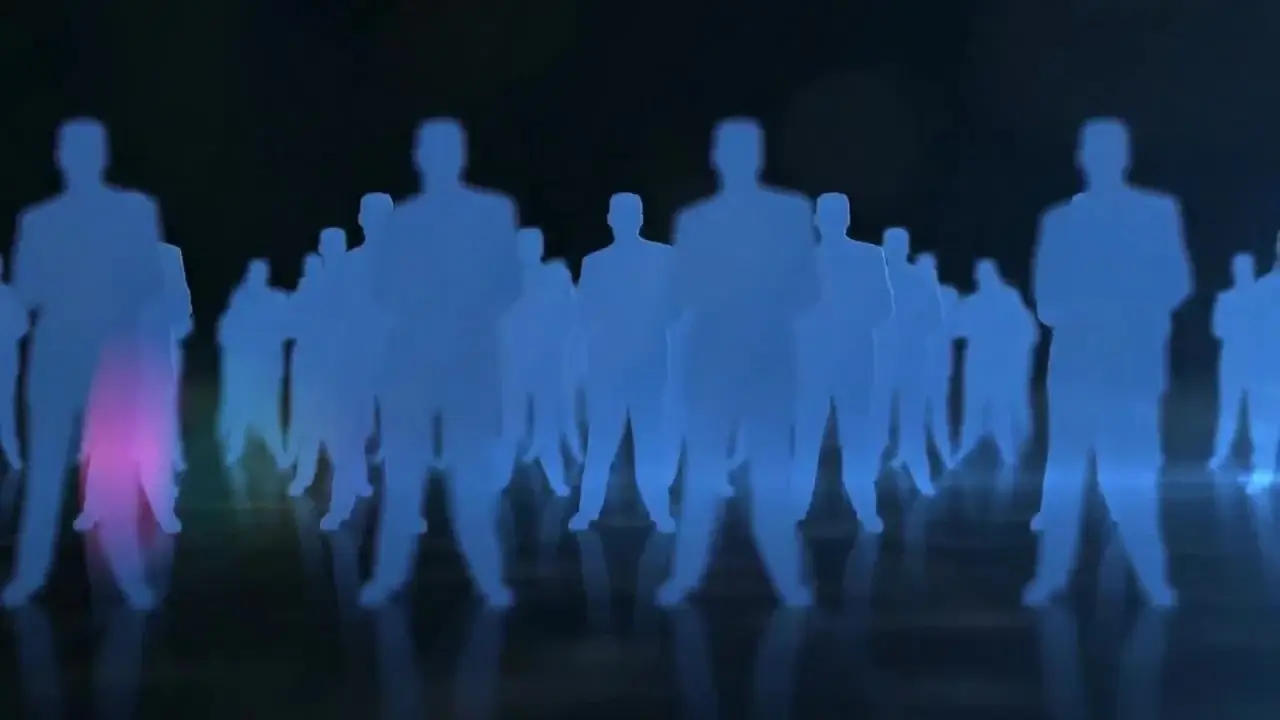
October 01, 2020
Synthetic Fraud Q&A with Socure’s Founder & CPO Johnny Ayers
How Socure Became the First Identity Verification Solution to Win IDC’s Future of Trust Award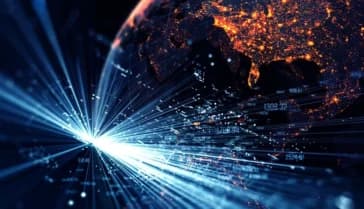
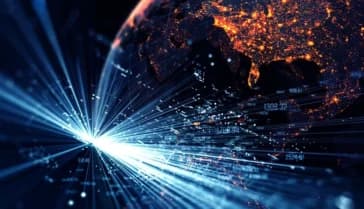
January 27, 2022
How Socure Became the First Identity Verification Solution to Win IDC’s Future of Trust Award
Addressing the Role of Synthetic Identity in P2P Fraud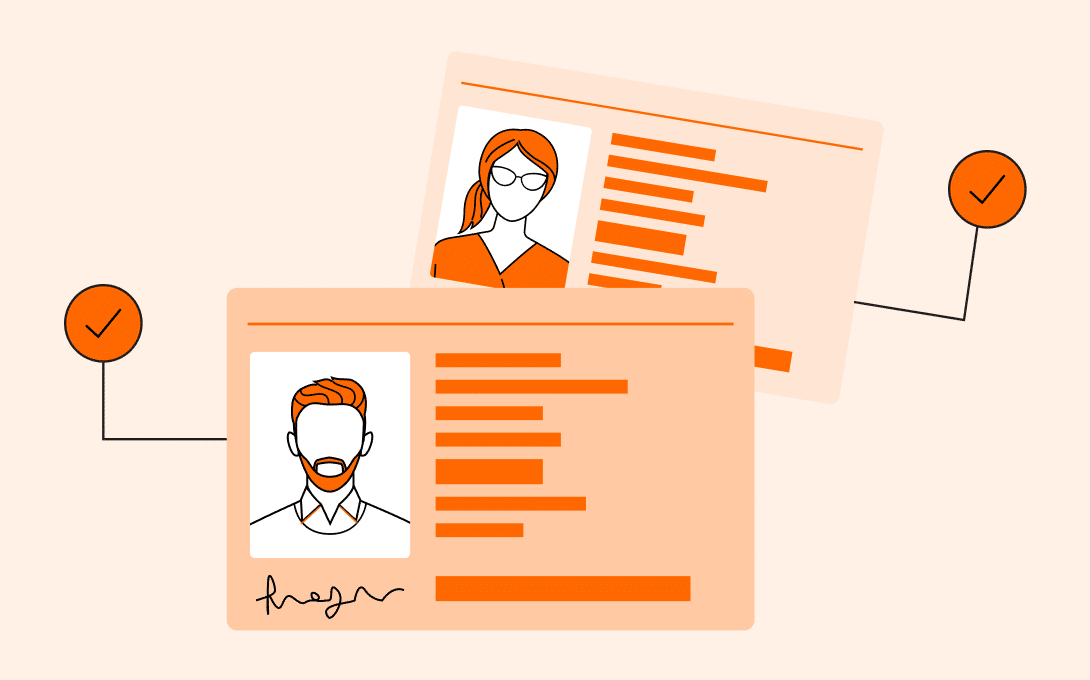
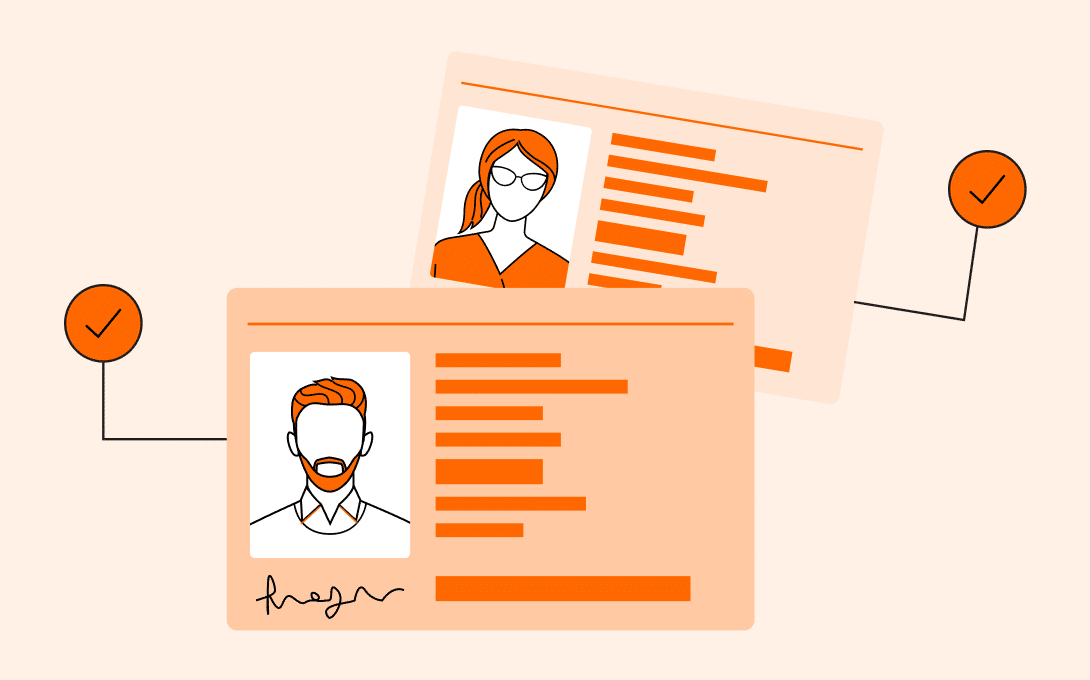
December 13, 2022
Addressing the Role of Synthetic Identity in P2P Fraud