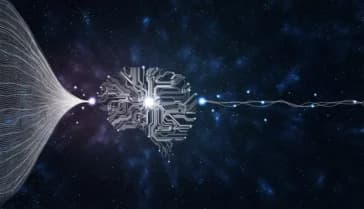
September 23, 2021
3 Reasons Machine Learning is Key to Predictive Document Verification
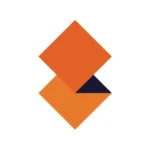
Socure
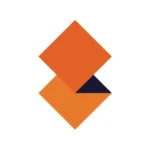
Socure
Socure is the leading platform for digital identity verification and trust. Its predictive analytics platform applies artificial intelligence and machine learning techniques with trusted online/offline data intelligence from email, phone, address, IP, device, velocity, and the broader internet to verify identities in real time.
Related Posts
3 Common Digital ID Verification Pain Points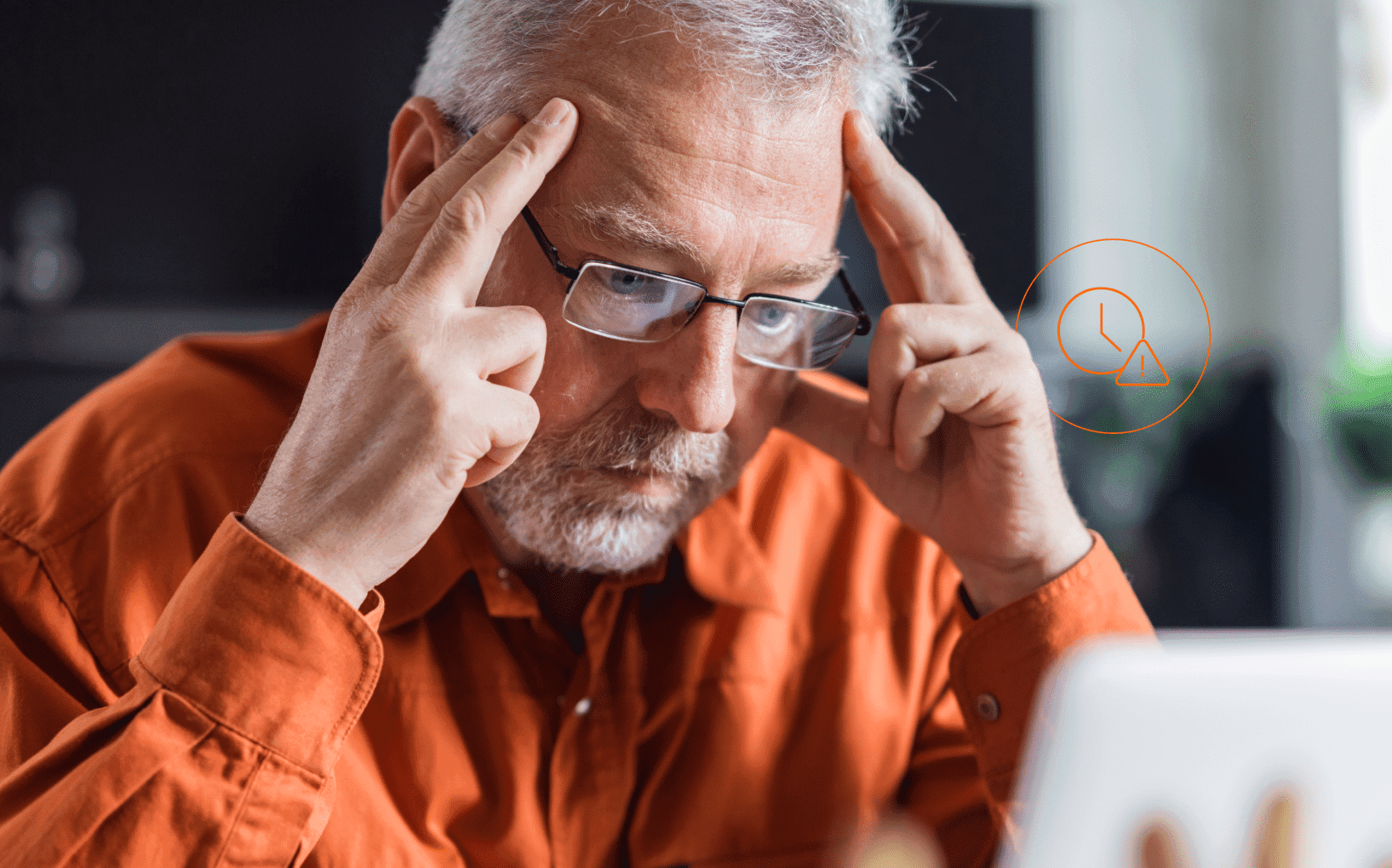
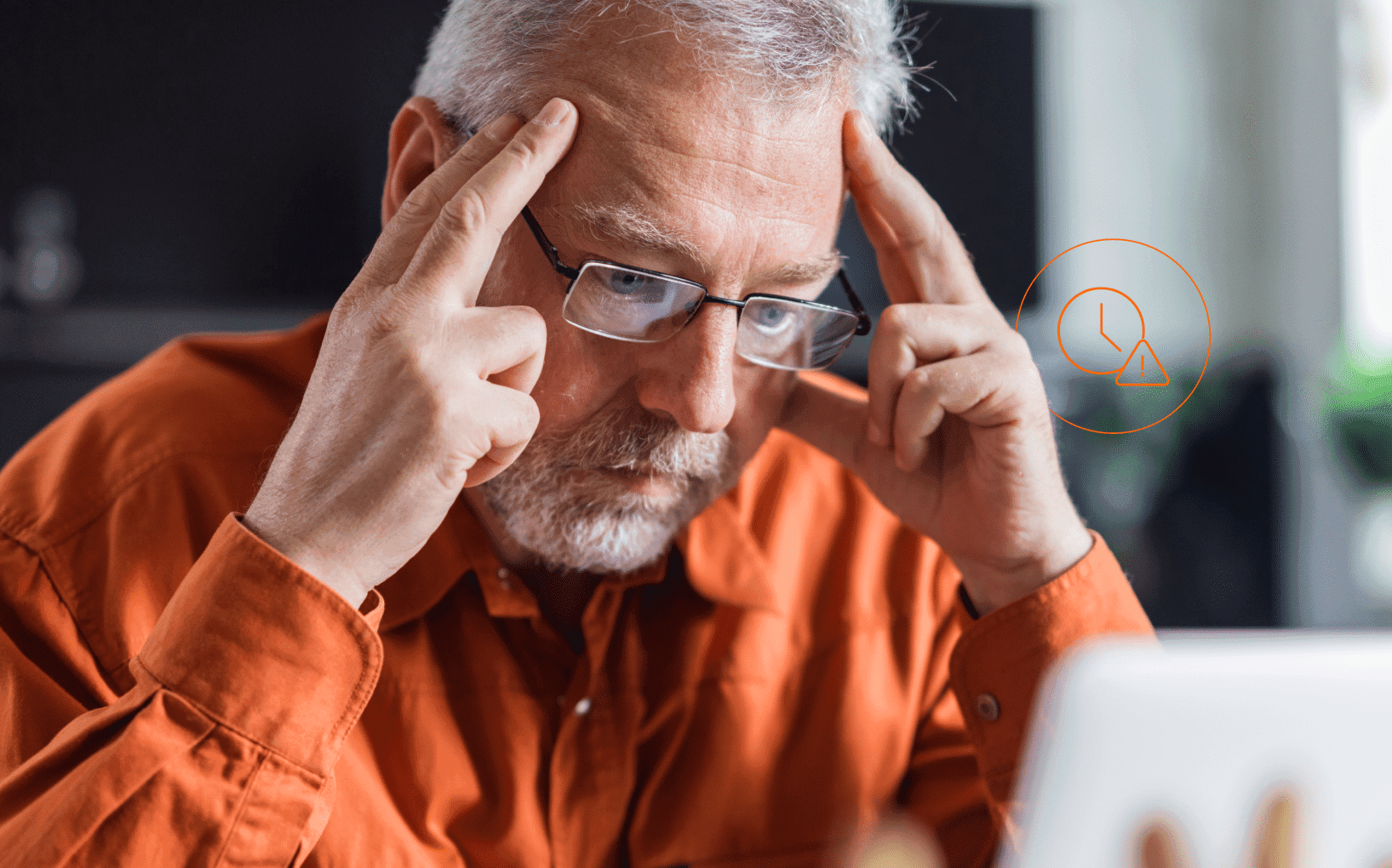
February 14, 2022
3 Common Digital ID Verification Pain Points
Account Validation Solutions: Which One is Best for Your Organization?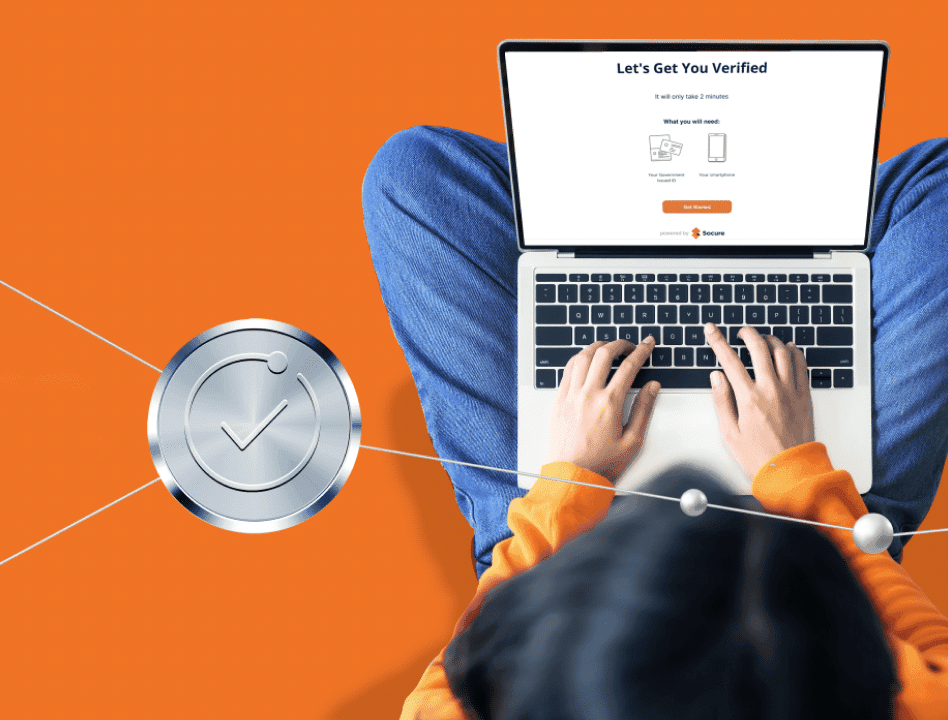
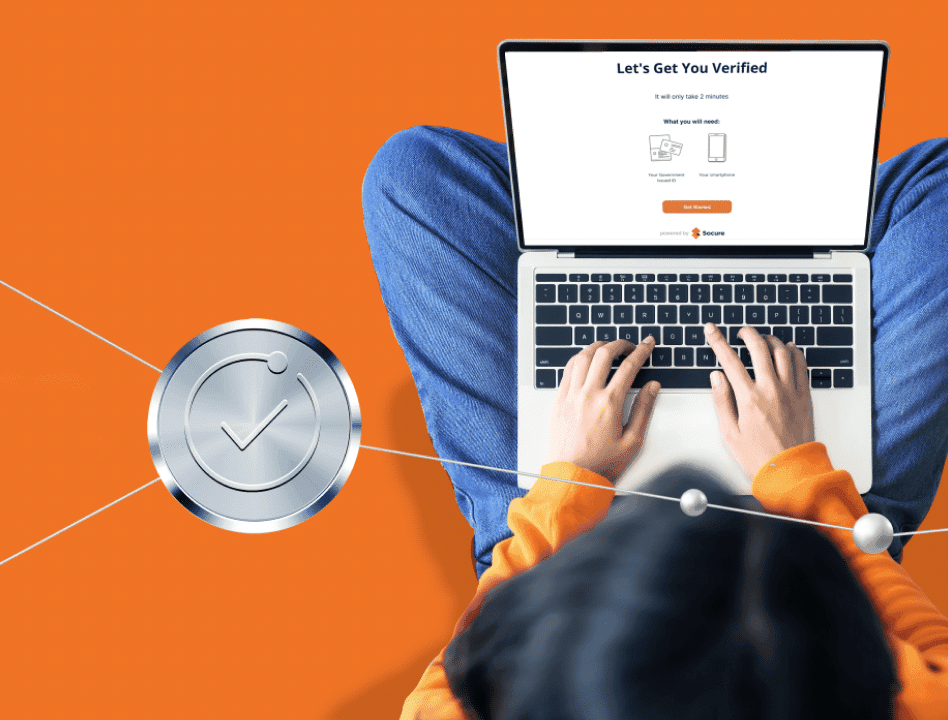
March 08, 2023
Account Validation Solutions: Which One is Best for Your Organization?